Google takes down AI image Generator due to its accused “woke” results. The release of the language-based model has resulted in utter criticism for releasing images that don’t tie back to the “accurate” depiction of white people in history.
Elon Musk took to X, formerly known as Twitter to post the tool is “woke”. The images are a reflection of its creators.
The general public is in an uproar with Gemini creating images of “diverse” Nazi generals and people of color as wartime heroes of American History. Apparently, the images generated are not aligned with the history taught in schools and recorded in books.
Googles response went something like this: Well if you asked for a “white” or “black” or “indigenous” teacher, astronaut it will give you a more accurate image of your request.
Excuse me? I’m sorry what?
Let’s start with what an a multimodal language model- such as Gemini actual consists of.
What exactly is a multimodal language AI Model?
A multimodal large language model is a sophisticated artificial intelligence system that integrates information from multiple modalities, such as text, images, and possibly other forms of data like audio or video.
This type of model goes beyond traditional language-only models by understanding and generating content in various formats. Multimodal models process and generate information in a more comprehensive and contextually rich manner.
By leveraging the combined power of language and visual understanding, multimodal large language models can perform tasks that require a deeper grasp of both textual and visual elements, enhancing their ability to comprehend and generate content across diverse contexts and applications.
These models are trained on vast datasets to learn complex patterns and relationships. It enables them to provide more nuanced and versatile responses across different types of input data.
What Steps Are Taken To Train a Multimodal Language Model?
Training a multimodal language model involves combining data from different modalities, such as text and images. Tools like Gemini use a large-scale dataset to teach the model to understand the relationships between images and text. Here’s a simplified overview of the process:
- Data Collection: Gather a diverse dataset containing pairs of text and corresponding images. This dataset should cover a wide range of topics and contexts to ensure the model learns robust representations.
- Preprocessing: Clean and preprocess the text and images. Tokenize the text and convert the images into a format suitable for the model. This may involve resizing, normalization, and other image processing techniques.
- Model Architecture: Design a multimodal architecture that can effectively process both textual and visual information. This typically involves combining a language model (for text) and a vision model (for images) and connecting them in a way that facilitates joint understanding.
- Pretraining: Pretrain the model on a large-scale multimodal dataset. During pretraining, the model learns general representations of both text and images. This phase might involve self-supervised learning, where the model predicts missing parts of the input or other auxiliary tasks.
- Fine-Tuning: Fine-tune the model on a specific task or domain using a task-specific dataset. This helps the model adapt its learned representations to the nuances of the target application, improving performance on particular multimodal tasks.
- Evaluation and Iteration: Evaluate the model’s performance on relevant benchmarks and real-world scenarios. Iterate on the model architecture, hyperparameters, and training process based on feedback and performance metrics.
- Deployment: Once satisfied with the model’s performance, deploy it for inference on new, unseen data in the target domain.
It’s crucial to note that training multimodal language models requires significant computational resources and expertise. Researchers and organizations often use large-scale GPU or TPU clusters to train these models efficiently. Additionally, careful consideration should be given to ethical considerations, bias mitigation, and data privacy throughout the entire process.
This list is not the only thing that goes into model training. However, it is a diligent list of what the back end looks like.
Why Is This Important?
Google’s team of engineers diligently trained Gemini utilizing extensive volumes of data. As such, a proactive approach should have been taken to evaluate the outcomes and determine its success or failure accordingly.
Based on my extensive experience in training AI tools, understanding what transpires within the so-called “black box”, once all the algorithms and datasets are amalgamated, remains elusive. Presently with the stringent programming parameters in place, Google’s staff has an environment conducive to testing and achieving desired results from this tool.
However, it seems they potentially overlooked an integral aspect: how their broader audience would interact with this instrument. This won’t always comprise engineers or stakeholders constantly aiming for 100% rates of success; it will predominantly include common users who may utilize it differently.
For instance, when formulating image-creating models – where each photo used for training the tool must be individually labelled as “white man,” “man with dog” or “little girl walking to school.”
Imaging Resource to Read:
In her enlightening book Unmasking AI Dr Joy Buloamwini refines upon potential hazards linked with facial recognition technology. She investigates the pitfalls stemming from incorrect labelling during AI model preparation. While her main focus is on human face detection systems there are clear parallels that can be drawn across other visual generative tools too. In fact, according to her assessment – issues associated with image datasets and how they’re labelled constitute a considerable part of these challenges.
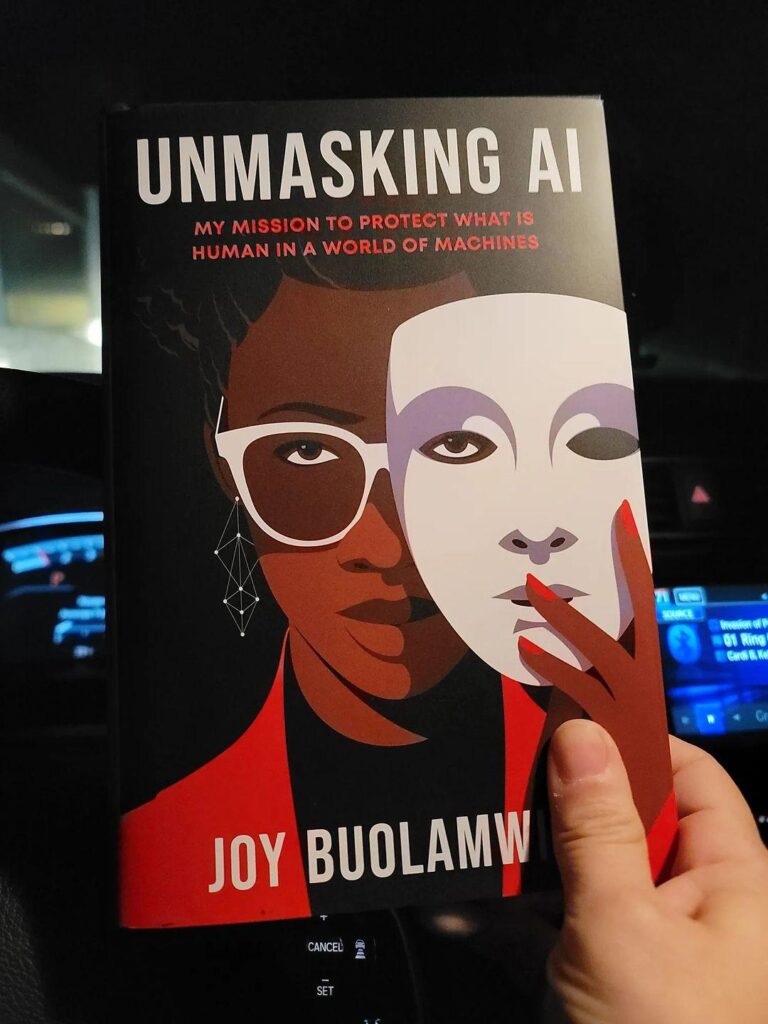
How the internet should have responded to Gemini and its images?
I am part of the internet and personally I thought it was hilarious.
Think about it – this gigantic company who has placed itself ahead of the AI race in saying we have this tool that can generate AI images with X accuracy. Gemni in fact made images that may not be historically accurate but captures the racial make up if the world. Who gets to define what is historically correct? Google? AI? The engineers who programed the tool?
Let’s also be realistic, none of the real time users of Gemini were present when history was taking place. They were not present when it was recorded via verbal or paper so can we really say those images are not accurate? We all know what happens when we play the game of telephone. The original message is not what is being communicated at the end of the line.
Can we really say there wasn’t a black general in the 1800’s? I mean black bodies were used for everything else. Their accomplishments erased and replaced with white bodies. Who’s to say that Gemini is actually wrong?
Was the real purpose of launching Gemini in the first place all about historical accuracy? I put my money on profitability and not accuracy.
Take away: everything is political. Google is part of the big five that is allegedly helping congress create safe AI tools.